AI vs. MD: Understanding the Limits of Digital Diagnosis
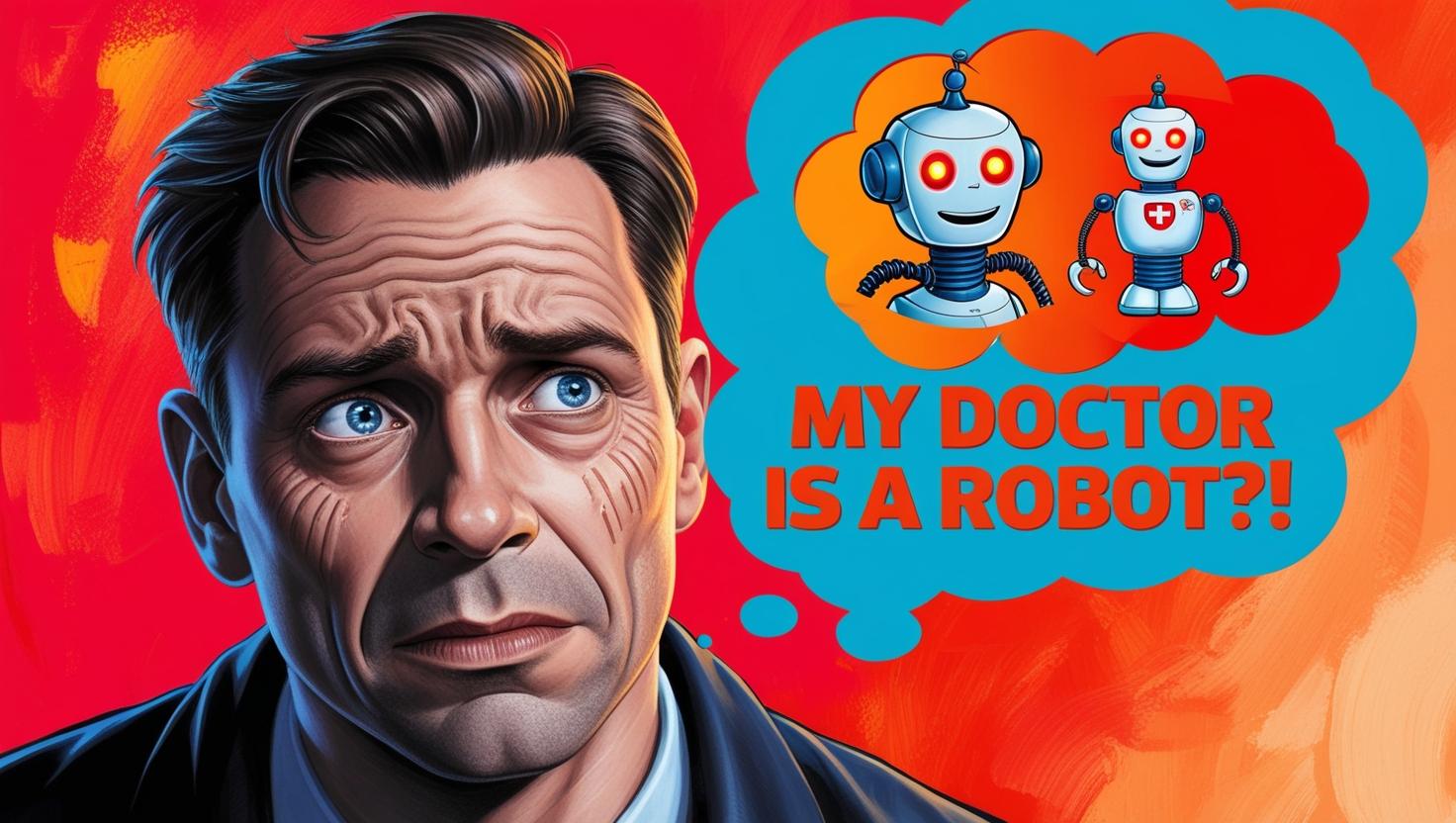
Artificial Intelligence (AI) is increasingly being touted as a revolutionary force, promising to enhance diagnostic accuracy, streamline processes, and ultimately improve patient outcomes. However, beneath the surface of this technological promise lies a complex web of limitations, ethical considerations, and potential pitfalls. In this blog post, we'll delve into the world of AI in medical diagnosis, comparing its capabilities to those of human doctors, exploring its inherent weaknesses, and emphasizing the crucial role of human clinical observation. Join us as we navigate the exciting, yet sometimes unsettling, intersection of AI and healthcare. Be sure to listen to our latest episode, When Should You Trust a Machine With Your Life?, where we explore this topic in even greater detail!
Introduction: The Rise of AI in Healthcare
The integration of AI into healthcare is no longer a futuristic fantasy; it's a present-day reality. AI algorithms are being developed and deployed across various medical domains, from radiology and pathology to drug discovery and personalized medicine. Proponents of AI in healthcare emphasize its potential to analyze vast amounts of data with unparalleled speed and accuracy, identify patterns and anomalies that might be missed by human clinicians, and ultimately improve diagnostic precision and treatment effectiveness. Indeed, studies show AI can reach impressive accuracy rates in certain diagnostic tasks. But is that enough to replace your doctor?
AI vs. MD: A Closer Look at Diagnostic Accuracy
When it comes to diagnostic accuracy, AI has demonstrated impressive results in specific areas. For example, AI algorithms have shown remarkable proficiency in detecting cancerous tumors in medical images, such as mammograms and CT scans. Some studies suggest that AI can even surpass human radiologists in identifying subtle signs of malignancy. A statistic we discussed in our episode showed that AI can be around 77% accurate, while a doctor is roughly 66% accurate, however, that statistic is very misleading. The algorithms that are being used are only as good as the data they are being trained on, so you can not assume that those results are consistent across the board.
However, it's important to approach these findings with a healthy dose of skepticism. Diagnostic accuracy is not the sole determinant of effective healthcare. While AI may excel in identifying specific patterns or anomalies, it often lacks the contextual understanding, clinical judgment, and empathy that are essential components of human medical practice. Human doctors are trained to consider a wide range of factors, including patient history, physical examination findings, and psychosocial context, when making diagnostic and treatment decisions. AI, on the other hand, typically relies on predefined algorithms and datasets, which may not fully capture the complexity of human health.
The Limitations of AI in Medical Diagnosis
Despite its potential, AI in medical diagnosis faces several significant limitations. One of the most critical limitations is the lack of generalizability. AI algorithms are typically trained on specific datasets, which may not be representative of the broader patient population. As a result, an AI algorithm that performs well in one clinical setting may fail to deliver accurate results in another setting with different patient demographics, disease prevalence, or diagnostic protocols. This issue is further compounded by the fact that many AI datasets are biased, reflecting the historical underrepresentation of certain racial and ethnic groups in medical research.
Another limitation of AI is its inability to handle ambiguous or incomplete data effectively. In real-world clinical practice, doctors often encounter patients with vague symptoms, atypical presentations, or complex medical histories. Human clinicians are trained to use their clinical judgment and reasoning skills to navigate these uncertainties and arrive at a diagnosis. AI algorithms, on the other hand, may struggle to make accurate predictions when faced with ambiguous or incomplete data, potentially leading to misdiagnosis or delayed treatment.
The Dangers of Over-Reliance on AI Self-Diagnosis
The increasing accessibility of AI-powered diagnostic tools raises concerns about the potential for over-reliance on self-diagnosis. While AI may offer convenient access to medical information and preliminary assessments, it's crucial to recognize that self-diagnosis based solely on AI algorithms can be dangerous. AI algorithms are not designed to replace human doctors, and they should not be used as a substitute for professional medical advice. Self-diagnosis based on AI may lead to inaccurate conclusions, delayed treatment, and unnecessary anxiety.
Moreover, self-diagnosis based on AI can exacerbate existing health disparities. Individuals with limited access to healthcare or health literacy may be more likely to rely on AI for self-diagnosis, potentially leading to delayed or inadequate treatment. It's essential to ensure that AI-powered diagnostic tools are used responsibly and ethically, with appropriate safeguards to prevent over-reliance and promote access to professional medical care.
Regional and Seasonal Blind Spots of AI
One of the key points we touched on in our latest episode is that AI struggles to account for regional and seasonal factors that can significantly impact medical diagnoses. For example, an AI algorithm trained to diagnose respiratory illnesses may not be able to differentiate between seasonal allergies and a parasitic infection that is common in specific geographic regions. This is because the algorithm may not have been trained on data that reflects the unique environmental and epidemiological conditions of those regions.
Human doctors, on the other hand, are trained to consider regional and seasonal factors when making diagnoses. They are aware of the prevalence of certain diseases in their local area and are able to adjust their diagnostic approach accordingly. This contextual awareness is crucial for accurate diagnosis, particularly in areas with diverse environmental conditions or seasonal disease patterns. An AI might misdiagnose Lyme disease symptoms as the flu in areas where Lyme disease is not prevalent, completely missing a crucial element.
The Role of Insurance Companies and AI-Driven Cost Minimization
The increasing adoption of AI in healthcare also raises concerns about the potential for insurance companies to use AI to minimize costs and deny coverage. AI algorithms can be used to analyze patient data, predict healthcare utilization patterns, and identify individuals who are at high risk of developing chronic conditions. While this information can be used to improve care coordination and disease management, it can also be used to justify denial of coverage or limit access to expensive treatments.
For example, an insurance company might use AI to identify patients who are likely to require costly medical interventions in the future and deny them coverage based on their risk profile. This practice, known as "risk selection," can undermine the principles of universal healthcare and exacerbate existing health disparities. It's essential to establish clear ethical guidelines and regulatory safeguards to prevent insurance companies from using AI to discriminate against patients or deny them access to necessary medical care.
AI as a Mental Health Support Tool: Bridging the Gap
Despite the risks associated with over-reliance on AI for medical diagnosis, AI can also be a valuable tool for supporting mental health. AI-powered chatbots and virtual therapists can provide individuals with access to mental health support and resources between therapy appointments. These tools can offer a convenient and affordable way for individuals to manage their symptoms, track their progress, and connect with mental health professionals when needed.
However, it's crucial to recognize that AI-powered mental health tools are not a substitute for human therapists. AI algorithms are not capable of providing the same level of empathy, understanding, and therapeutic support as a human clinician. These tools should be used as a complement to, rather than a replacement for, traditional mental health treatment.
The Indispensable Value of Human Clinical Observation
In the era of AI, it's easy to overlook the indispensable value of human clinical observation. Human doctors possess a unique ability to observe patients, listen to their concerns, and gather information that may not be readily available in medical records or diagnostic tests. This clinical intuition, honed through years of experience and training, is essential for accurate diagnosis, particularly in cases involving rare or unusual conditions. A machine might not be able to factor in the look in someone's eyes or pick up on the nuances of their speech.
Human doctors are also able to build rapport with patients, establishing trust and fostering open communication. This therapeutic relationship is crucial for effective treatment, as it allows patients to feel comfortable sharing their concerns and adhering to treatment recommendations. AI algorithms, on the other hand, are unable to replicate this human connection, which can limit their effectiveness in certain clinical settings.
The Risk of Depersonalization in Automated Healthcare
As healthcare becomes increasingly automated, there is a risk of depersonalization. The increasing reliance on AI algorithms and digital technologies can lead to a decline in human interaction between doctors and patients. This depersonalization can erode the therapeutic relationship, reduce patient satisfaction, and ultimately compromise the quality of care.
It's essential to ensure that AI is used in a way that complements, rather than replaces, human interaction in healthcare. Technology should be used to enhance the efficiency and effectiveness of medical care, but it should not come at the expense of human connection and empathy. Doctors should continue to prioritize face-to-face interactions with patients, taking the time to listen to their concerns and provide personalized care.
AI's Struggle with Complex Medical Data Interpretation
While AI can excel at analyzing large datasets, it often struggles with the nuances of complex medical data interpretation. Medical data, such as blood work, lab results, and imaging studies, often contain subtle patterns and anomalies that are difficult for AI algorithms to detect. Human doctors, on the other hand, are trained to interpret these data in the context of the patient's overall clinical presentation, taking into account factors such as age, gender, medical history, and lifestyle.
Moreover, medical data is often incomplete or inaccurate, which can further complicate the interpretation process. Human doctors are able to use their clinical judgment and reasoning skills to identify and correct errors in medical data, ensuring that diagnostic and treatment decisions are based on accurate information. AI algorithms, on the other hand, may be more susceptible to errors in medical data, potentially leading to inaccurate conclusions.
The 'Google Effect' and Confirmation Bias in Self-Diagnosis
The ease with which people can access medical information online has led to the "Google effect," where individuals increasingly rely on online search engines to self-diagnose their medical conditions. While online medical information can be a valuable resource, it can also be misleading or inaccurate. Moreover, individuals who self-diagnose based on online information are often subject to confirmation bias, selectively seeking out information that confirms their pre-existing beliefs about their health.
This confirmation bias can lead to inaccurate self-diagnosis and delayed treatment. Individuals may dismiss or downplay symptoms that do not align with their preconceived notions, potentially leading to a delay in seeking professional medical care. It's essential to approach online medical information with a critical eye and to consult with a qualified healthcare professional for accurate diagnosis and treatment.
Collaborative Approaches: AI and Human Medical Professionals Working Together
The future of healthcare likely lies in collaborative approaches, where AI and human medical professionals work together to deliver the best possible care. AI can be used to augment human capabilities, providing doctors with valuable insights and decision support tools. However, AI should not be viewed as a replacement for human doctors, but rather as a tool to enhance their efficiency and effectiveness. We covered this point in detail in our most recent episode, and believe it's critical to getting the best results.
For example, AI can be used to analyze medical images and identify potential abnormalities, freeing up radiologists to focus on more complex cases. AI can also be used to personalize treatment plans based on individual patient characteristics, ensuring that patients receive the most effective and appropriate care. By combining the strengths of AI and human intelligence, we can create a healthcare system that is both efficient and compassionate.
Conclusion: Finding the Right Balance Between AI and Human Expertise
AI has the potential to revolutionize healthcare, but it's essential to approach this technology with caution and recognize its limitations. AI should not be viewed as a replacement for human doctors, but rather as a tool to enhance their capabilities and improve patient outcomes. We must find the right balance between AI and human expertise, ensuring that technology is used in a way that complements, rather than replaces, human interaction and clinical judgment.
Ultimately, the goal of healthcare is to improve the health and well-being of individuals. AI can play a valuable role in achieving this goal, but it's crucial to ensure that technology is used ethically and responsibly, with appropriate safeguards to protect patient rights and promote access to quality medical care. To dive deeper into this discussion, be sure to listen to our episode When Should You Trust a Machine With Your Life?. We hope this blog post has provided you with a more nuanced understanding of the role of AI in medical diagnosis and the importance of human clinical observation.